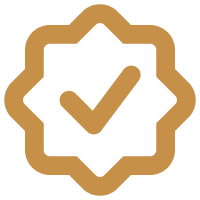
More precise detection of wafer/chip defects
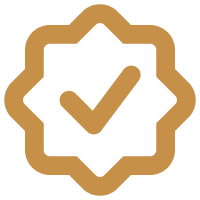
Automated classification of defect types
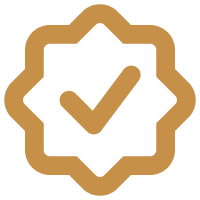
Stronger pattern defect detection capabilities
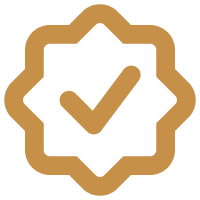
Significantly improved detection efficiency
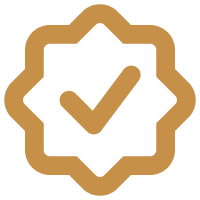
Reduced human intervention and subjective errors
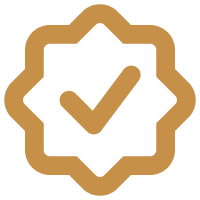
Enhancing defect detection for light guide plates and diffusion boards
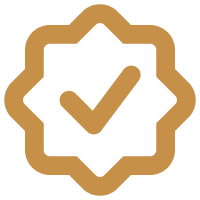
Accurate detection of fine circuit pattern defects
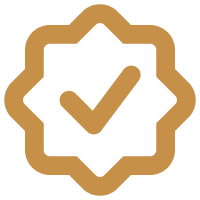
Improved detection rate for color filter and CF defects
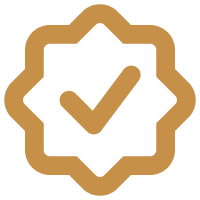
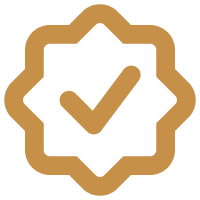
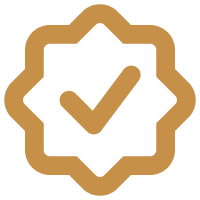
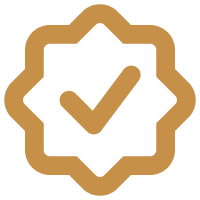
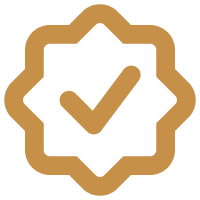
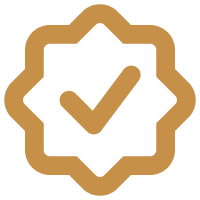
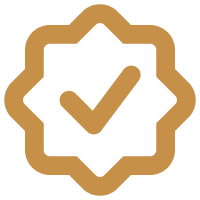
Enhancing defect detection for complex metal/mechanical components
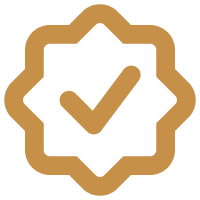
Handling diverse metal materials and surface characteristics
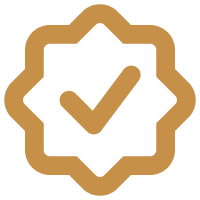
Improving defect detection for critical functional components
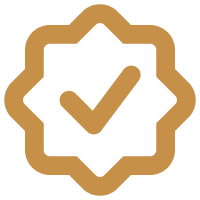
Shortening programming time for complex product inspections
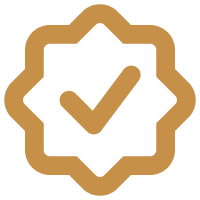
Enhancing high-speed production line inspection adaptability
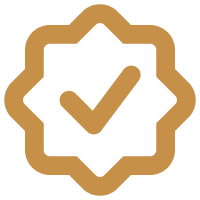
Real-time problem identification and resolution
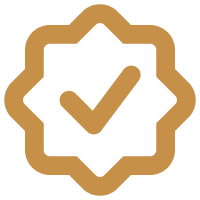
Reducing human errors
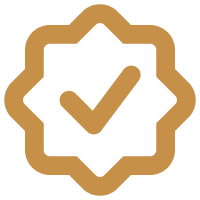
Reducing customer complaints and increasing ROI
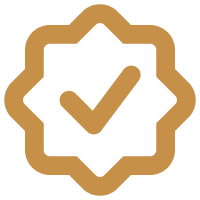
Lowering production costs
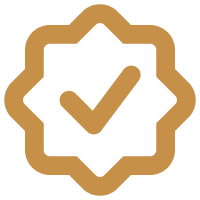
More precise detection of food surface defects
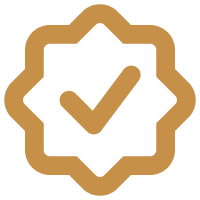
Accurate identification of foreign objects within food
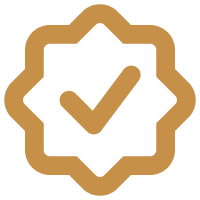
Automated food shape recognition and grading
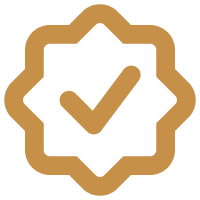
Rapid inspection of food packaging integrity
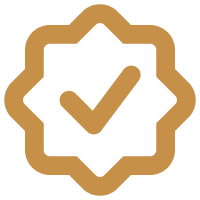
Improved detection of subtle surface defects on medical devices
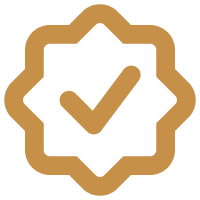
Accurate inspection of medical packaging integrity
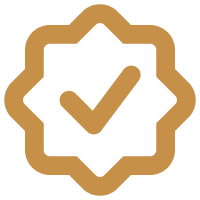
Realization of medical label character recognition
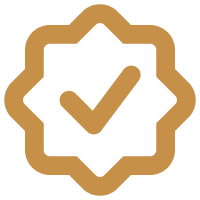
Improved inspection efficiency and reduced human risks
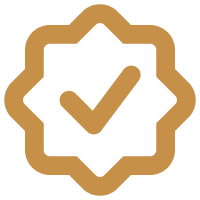
Improved detection accuracy
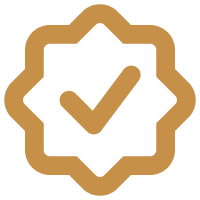
Flexible defect definition and classification
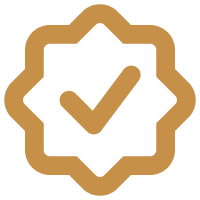
Highly adaptive optimization of inspection
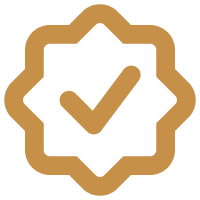
Automated, unattended operation
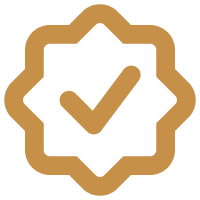
Improved detection of small defects on printed products
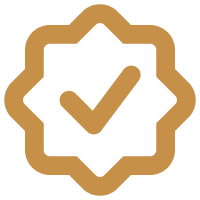
Accurate text/code recognition Labels/printed products often feature text, barcodes, and other encoded information.
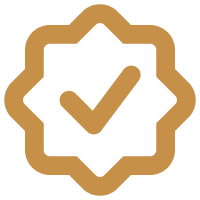
Efficient inspection of complex patterns and image defects
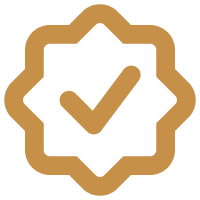
Significantly improved inspection efficiency
Food
Solutions:
The In-Sight 3D-L4000's unique optical scanning engine also offers several advantages over other competing solutions, including eye-safe operation, while providing more light to the surface to increase throughput, providing more accurate 3D point clouds for measurement values, and even 100% of the In-Sight 3D-L4000's field of view can be measured even if debris blocks 50% of the laser.
Cognex Deep Learning is ideal for visually inspecting containers for defects before they are sealed and shipped. The defect detection tool can be trained on a small set of images of cartons properly filled with the correct number of tea bags. The defect detection tool can then identify any anomalies in the carton, including misalignment, quantity, orientation, or errors in visible strings and labels. The identified cartons can then be sent back to correct the errors and repackaged.
- Cognex Deep Learning is ideal for the complex task of finding and picking good nuts that are oriented correctly and placing them on chocolate.
- The classification tool is trained on a set of images of good and bad nuts. The tool can quickly classify nuts into acceptable and unacceptable categories.
- The part location tool is trained on a set of images of good nuts that are oriented correctly. It can then locate the nuts as they pass by, regardless of the number of nuts present at the same time.
- Combining these tools ensures that good nuts are identified and located so that they can be picked and placed on the chocolate by a robotic arm. Manual inspection or other forms of machine vision cannot achieve the same speed and accuracy for this task.
Cognex robust modular In-Sight 7800 series machine vision systems tackle this application using multiple tools (histograms, edges, etc.) complemented by externally mounted linear lights above mouthpieces. In-Sight 7800 detects contrast disparities between mouthpiece and filter paper, validating presence and proper placement. Edged tools scrutinize recessed mouthpiece tail ends for incisions, segregating rejects from fast-paced production lines. Efficient installation follows suit, accompanied by simple configuration enabled via In-Sight Resource Manager software. Network-enabled Human-Machine Interfaces monitor test outcomes remotely.
Cognex Deep Learning can solve the orientation problem of liquid filling bags and other flexible containers during packaging and transportation. It can be trained on a set of images of possible containers with different orientation labels. The classification tool can accurately determine the orientation of each bag seen and provide the data to the picking and placing robots, which then pick and place them in the correct orientation in the secondary packaging containers.
Four AVT Prosilica GC1290C cameras are used to inspect raisins and sort them by appearance and size before packing them in boxes.
Cognex PatMax technology and color tools can identify designs and stamps, regardless of changes in direction, angle, lighting, and other factors that can affect the appearance of items on the production line. PatMax uses a set of boundary curves to learn the geometric shape of an object and then searches for similar shapes in the image, without relying on specific grayscale values. Damaged or broken products are removed before being packaged or shipped, avoiding costly returns and protecting brand reputation.
Cognex In-Sight 2800 Series Smart Cameras fit neatly into confined quarters of tobacco manufacturer gear. Mounted above hopper bins, In-Sight 2800 sports embedded high-powered lighting rigs, distinguishing loose tobacco apart from pouches. Surface Flaw Tools match perfectly with Filter Program Tools, highlighting luminosity and contrast discrepancies indicative of perforated states especially handy for similarly colored brown tobaccos. Dependably inspecting vessel contents, In-Sight 2800 removes faulty chewing tobacco packs prior to reaching consumers' hands.
Cognex 2D vision systems with edge learning perform pass/fail inspection and reject defective items or packages when detected. The embedded edge learning tool can be trained with just a few images that classify packages as pass (all items present) and fail (one or more items missing). Even under shrink wrap, it can confirm that all bottles and products are present, helping food and beverage manufacturers prevent operational errors and maintain customer satisfaction.
With simple drag-and-drop modules, you can easily create flowcharts to guide the arm for sorting and packaging.
Using the blob counting tool, the In-Sight 2000 sensor can detect the presence or absence of relevant liquids. When the bottle is underfilled, there will be too few blobs displayed, and when the bottle is overfilled, there will be too many. Bottles that fail the inspection will trigger the rejection function.
Cognex Deep Learning can easily confirm the presence and absence of straws without damage. It can be trained on a small set of images of undamaged straws, as well as a set of images of various unacceptable missing, damaged, or misplaced straws. The classification tool learns to ignore any background and classifies all images into acceptable or unacceptable states. Since there is no need to identify and define specific defects, the classification process is very fast. It only needs to judge acceptable/unacceptable.
Cognex Deep Learning can reliably detect these types of products to prevent defective items from reaching customers. It is trained on a set of images of good items and another set of images of items with various types of coating damage. The classification tool can then quickly classify chewing gum or other items into acceptable and unacceptable categories. This level of quality cannot be achieved with other types of visual inspection.
Foreign objects such as rubber bands, plastic, wire, machine parts, bone fragments, and fish bones can be generated during the food production process. In addition to damaging the brand's reputation, food hygiene complaints may also result in huge compensation claims. Anko has partnered with a cross-domain company to launch a food X-ray inspection machine that is suitable for the food, pharmaceutical, and chemical industries. It can detect tiny contaminants that are difficult to identify with the naked eye, such as wire, stainless steel shavings, and plastic sheets. It also has the function of checking the quantity and defects of food.
Cognex Deep Learning can reliably identify foreign objects, invalid seals, contamination, and many other problems that can affect product integrity. 100% vision inspection can reduce operator error and achieve maximum efficiency. Deep learning can also further highlight problems in real time, allowing operators or machines to clearly identify problems, which can be classified later.
Cognex deep learning can instantly locate, analyze, and classify complex contamination issues, preventing large-scale production line contaminants from entering the supply chain. Deep learning combines human-like detection capabilities with the automation, scalability, and repeatability of a computerized system. This capability can be further enhanced by using robots to ensure that machines work together with vision tools to detect the most complex anomalies that operators sometimes miss. The end result is a reduction in recalls, reduced rework costs, and complete product image capture and full traceability.
Cognex's In-Sight 9912 Visual System delivers automated solutions for each box complete with distinct labels, such as emblems, names, or numerical codes. Once cargo arrives near the camera, In-Sight 9912 utilizes PatMax object localization technology, instantly spotlighting selected marks or motifs prior to computing quantities contained therein. Its expansive field of view and optical arrangement make it ideally suited for massive crates and distant checkpoints. Displaying results on tablet computers or laptops, flatscreen monitors, or web-based HMIs empowers operators to review examination outcomes.
The compact yet powerful In-Sight 8000 vision system can detect e-cigarettes to help tobacco manufacturers ensure their products are free of harmful debris. The In-Sight 8402 can be easily mounted inside the machine with an external light bar to evenly illuminate the inspection area and check the filter. Since the region of interest is relatively small, reducing the field of view (FOV) of the vision system provides the 2MP In-Sight 8402 with the extra resolution needed to clearly identify defects while keeping up with high-speed machines. Like all In-Sight, the In-Sight 8000 series can be quickly and easily configured and deployed using In-Sight Explorer software with the intuitive EasyBuilder configuration environment.
- The component location tool can be trained on a small set of images of pizzas with the desired number of pepperoni slices or other toppings. It can then identify, locate, and count pepperoni slices, even if they are touching or overlapping, while ignoring variations in cheese and sauce underneath. If the number of toppings is not within the specified limits, the pizza will be rejected.
- The defect detection tool can be trained on a set of images of acceptable pizzas, even with multiple different toppings. It will then detect any physical contaminants, including toppings that are not suitable for this type of pizza, while ignoring variations in cheese and sauce underneath.
- The classification tool can be trained on a set of images of all types of pizzas produced in the factory, with labels. It can then distinguish between different variations and classify them so that they can be put into the appropriate packaging and accurately entered into inventory. These tools can be used all together to help manufacturers automate processed food inspection, ensuring that only the highest quality products leave the factory and end up on customers' kitchen tables.
Many modern 3D vision solutions offer simple and easy-to-use blob, volume, and edge detection tools to solve complex food inspection applications. The In-Sight 3D-L4000 smart camera, combined with Cognex In-Sight software, streamlines the process and makes these tools easy to use in a familiar spreadsheet format. The In-Sight 3D-L4000 offers the highest quality 2K resolution and a patented, no-spot blue laser optical configuration that generates more accurate 3D point clouds for inline measurements. Because the In-Sight 3D-L4000 can generate a complete 3D point cloud, rather than just a height map above the surface, it can more accurately apply 3D blob tools to identify different, but touching, objects, such as cookies in a container. The In-Sight 3D-L4000 also offers a variety of true 3D algorithms for volume measurements, such as the distance between cylinders.
Cognex Deep Learning is ideal for detecting small, unpredictable defects. It is trained on a set of chocolate images that includes the full range of acceptable variations. The defect detection tool then flags any chocolate that falls outside of this range, regardless of the type of defect. These must all be removed to maintain quality standards.
Cognex Deep Learning is ideal for sorting coffee beans because it can quickly and easily identify different colors, sizes, and characteristics of coffee beans. It can be trained on a set of images of each type of coffee bean that is received in the warehouse. Even if different types of beans look very similar, the classification tool can distinguish between different types, while accepting natural variations within each type. The defect detection tool can identify any physical contaminants in a batch of coffee beans before they are sent for blending.
Cognex AI image analysis software is as reliable as human inspectors for detecting surface appearance defects, but with the speed of a computerized system. The defect detection tool can create reliable shape and texture models based on training images, and can find defects on rough materials even with poor image quality under standard lighting. It then identifies surface texture changes as anomalies, and then uses the classification tool to classify them as collisions or scratches. Continuously providing high-quality products to the market can improve customer satisfaction and improve brand loyalty.
Employing Histogram Tools, In-Sight 7800 examines representative signs of poor packaging, including uneven brightness and absence of brightness whilst installed behind exterior linear lights. Such arrangements facilitate high resolution, swift processing, and broad fields of view vital for monitoring the rapid movement of cigarette rolling devices surrounding assembly wheels. An incorporated pan-tilt housing safeguards against debris originating from tobacco production machinery. Additionally, protected by IP67-rated lens covers, In-Sight 7800 ensures durable functionality. Configurable using intuitive and easy-to-deploy In-Sight Manager software, integration with factory automation environments remains straightforward.
Cognex In-Sight 2000 sensors guarantee correct cutting according to specified parameters, leveraging Edge Detection Tools to find either end of cigarette filters and pinpoint the center point for cigar cuttings. Boasting compact designs fitting snuggly into tight spaces available within tobacco equipment, In-Sight 2000 integrates potent built-in lighting delivering essential illumination coupled with multiple wide-angle camera options providing expansive views at close working distance ranges, capturing high-resolution images.
Cognex In-Sight 2000 sensors offer superior performance for dual inspections where space constraints necessitate diminutive dimensions - measuring just 92 x 60 x 52 mm. Powerful integrated lighting configurations deliver high-contrast, high-resolution images even at close proximity, suitable for checking filter paper alignments and dot glue detections. Upon discovery of defects, sensor transmits findings to programmable logic controllers (PLCs) promptly halting machinery operations, preventing resource squandering and blockages in tobacco production machines.
Cognex Deep Learning calculates the number of mouthpieces housed within large shipping cartons entirely, tackling this demanding application head-on. Merging positional instruments located overhead with million-pixel cameras and parallel placements of strong external linear lights, this approach counts transport containers holding over four thousand mouthpieces. Trained using a modest group of sample images, Cognex Deep Learning distinguishes among various mouthpiece defect categories, including white carbon, activated charcoal, concave structures, and others, ensuring accurate enumeration regardless of mouthpiece dimension, hue, or form.
Cognex AI-driven technologies identify and classify tears or holes appearing on cigarette boxes, assisting in the detection of potential production concerns threatening product quality.
- Cognex Deep Learning software can perform multiple feature location and identification tasks on a single image. Cognex Deep Learning classifies and distinguishes the functional features of different chocolates based on size, shape, and surface features.
- Users can train the location tool to locate each type of chocolate that needs to be found. Users can create a database of different types of chocolates for the location tool to locate, and then the location tool can be used to perform packaging verification.
- After training, the image can be segmented into different regions, and the location tool will be able to detect the presence of chocolate in these regions and verify that the type of chocolate is correct. Multiple configurations can also be created for different packaging variations on a single production line. In this way, users can automate the chocolate packaging verification process with just one tool.
Machine vision solutions can measure the volume of food dispensed during product assembly to ensure food portioning. 3D displacement sensors provide highly accurate 3D volume measurements specifically designed for food portioning. 3D calibration allows manufacturers to set food portioning and cut points during processing to ensure that portions are uniform and consistent. Cognex 3D displacement sensors are factory calibrated to detect 3D food products. The Cognex 3D laser displacement sensor can scan the dimensions of individual portions to determine if the portion meets the pre-programmed specifications. Visual inspection and volume measurement can be performed after scanning.
Cognex vision systems use multidimensional imaging to verify cap height and tilt. In-Sight vision systems use edge detection technology to measure the distance between the top of the cap and the neck of the bottle, and the horizontal position of the top of the cap, to confirm that it meets the pre-defined limits. In this way, the vision system can determine whether the cap is screwed on tightly and is in a safe sealed state. Bottles that fail the cap height and tilt inspection will be rejected.
In addition to solving the original packaging direction and counting problems of the manufacturer, this vision inspection system also identifies many other errors, including: juice box skew, missing, crushed, dropped, rotated or redundant, missing products after whole box packaging , inverted or inverted, damaged labels, etc.
Using Solomon's core AI machine vision system SolVision, an AI model can quickly and accurately identify the direction and angle of each artichoke, using its stem as a reference point, regardless of the size of the stem itself. SolVision also allows AI detection results to be exported through various communication protocols.
- Cognex AI vision systems and software are effective solutions for meat quality inspection. They can be trained on a set of images of each labeled cut of meat or poultry. The classification tool then accepts a range of natural variations while accurately classifying each cut, ensuring it receives the proper grade so that the product can be sold at the highest reasonable price.
- Regardless of the source, whether from damaged packaging, machinery, or other sources, the defect detection tool quickly finds any physical contaminants and flags them before the product is shipped. This ensures that all shipped cuts are correctly classified and priced, leading to increased customer satisfaction and revenue.
Cognex machine vision conducts cigarette package assessments, supporting tobacco producers in averting sales of defective or empty packages. Installed adjacent to rotational rollers, In-Sight 7800 executes surveillance on rapidly shifting cellophane wraps, triggering cessation upon overwrap loss or tearing occurrences. Combining integrated lighting with white LED arrays, optimum system performance emerges naturally sans additional expenses linked to pricey auxiliary lighting sources. Applying distribution graphs and contrast settings within In-Sight Resource Manager software, In-Sight 7800 readily solves this particular application.
Solomon combines machine vision and artificial intelligence to use the Segmentation technology of Solomon SolVision AI image platform to perform defect detection (Defect Identification). In the fast and mass production of fried food processing line, it can identify multiple different defect patterns and instantly detect defective products.
- The Cognex In-Sight 3D-L4000, combined with In-Sight software and 3D vision tools, can quickly complete metal can quality inspection, reduce rework and improve productivity.
- Both 2D and 3D vision are based on point cloud measurements, not just height maps. The In-Sight 3D-L4000 can measure the distance between blobs and features for advanced machine vision applications, achieving higher accuracy than traditional laser scanning solutions. Due to its advanced optical design and the ability to easily program in the In-Sight software environment, which includes 3D edge finding, 3D blob analysis, plane finding and many other functions, it also offers other advantages such as superior performance and safety.
After checking that the product level and cap position are correct, the vision system will detect the closure status to verify the seal integrity, and it will also specifically verify the presence of the tamper-evident band and the security seal. The machine vision system can detect defects and confirm the integrity of the packaging through accurate and consistent detection. The 3D laser displacement sensor ensures that the security seal covers the entire opening, there are no bubbles or wrinkles around the edge of the lid, and there are no holes or perforations. Machine vision technology can detect the edges of the security seal and measure its height, width, relative position and gap size (if any), and compare it with the pre-defined limits. Tamper-evident seals that do not meet the pre-defined limits will be rejected to reduce the risk of product recalls.
By using the Segmentation technology of SolVision AI image platform, the location of the crack defects in the image samples is located and labeled, and the AI model is trained based on this. After the training is completed, the AI can be used to detect the pores and cracks on the eggshell surface and then grade them, which can improve the safety and commercial value of egg consumption.
Different flavors of coffee are distinguished by capsules of different colors. One plate of coffee capsules is used as the standard group, and the other plate of coffee capsules is used as the control group. Using SolVision's Feature Detection tool, the images of each coffee capsule are learned. If any coffee capsule is placed incorrectly in the plate, SolVision can immediately detect the error and mark it with a box. It can successfully identify coffee capsules with high reflection and small color difference.